AI made 100x easier!
Langflow is the open source, visual framework for GenAI RAG apps. Deploy in minutes.
You can now focus on what you do best — developing your apps.
Generative AI Leaders Shaping Their Industries
One-stop GenAI Stack
A RAG API with all the data, tools, and an opinionated stack that just works. Both vector and structured data, secure, compliant, scalable, and supported. Integrated with LangChain, Vercel, GitHub Copilot and AI ecosystem leaders.
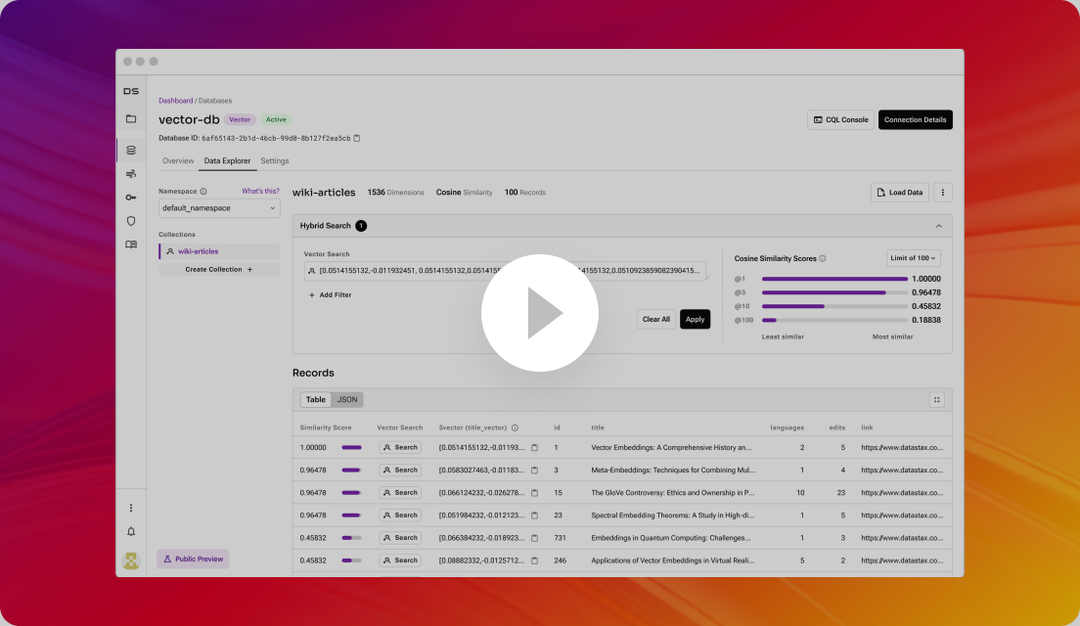
Relevant GenAI FTW
Minimize hallucinations with up to 20% higher relevance, 74x faster response time, and 9x higher throughput than Pinecone all at 80% lower TCO. Read while indexing to make data updates available with zero delay.
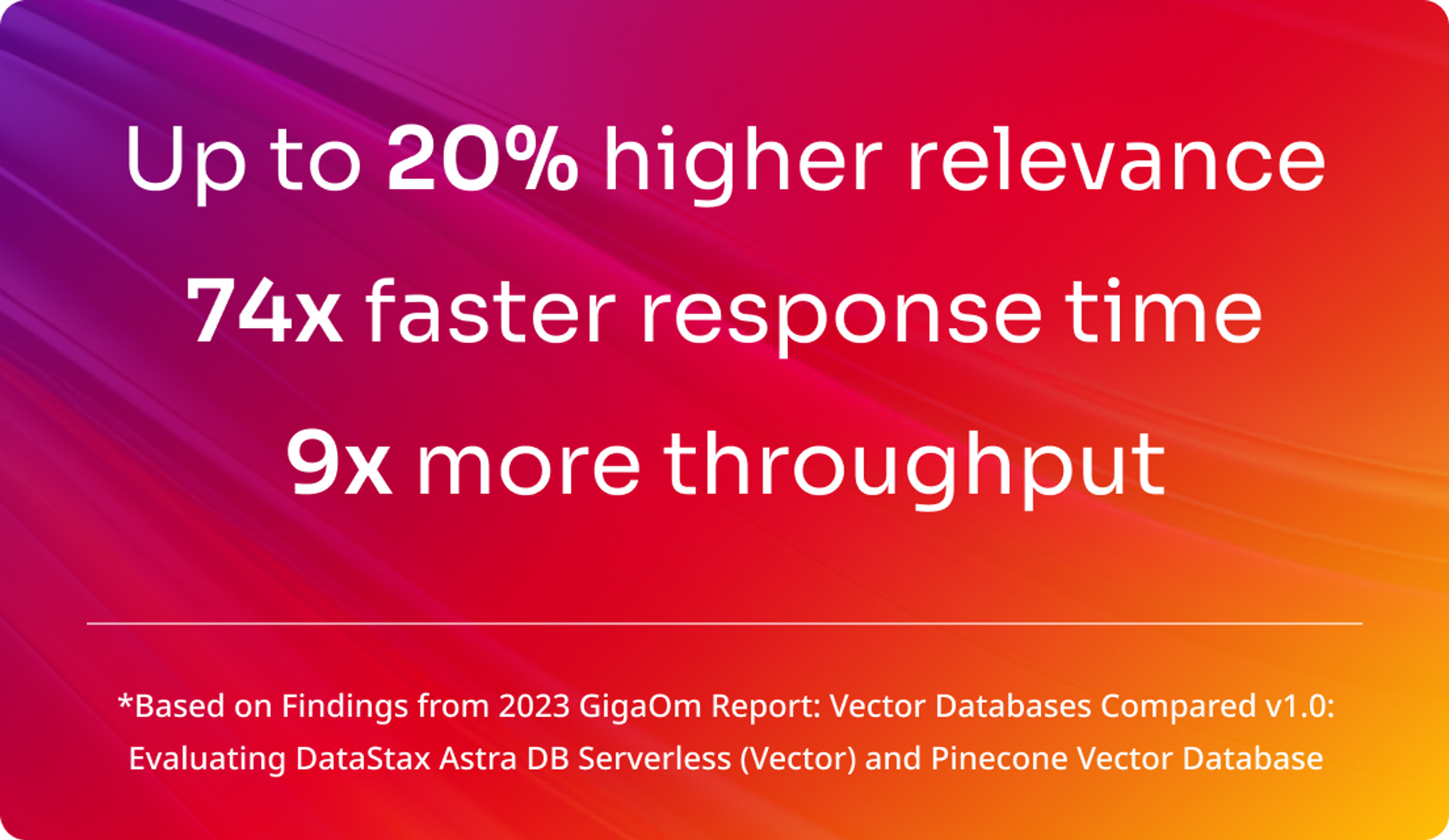
Fast Path to Production
Quickly take your GenAI idea into production. Deploy on the leader in production AI workloads and support global-scale on any cloud with enterprise level security and compliance.
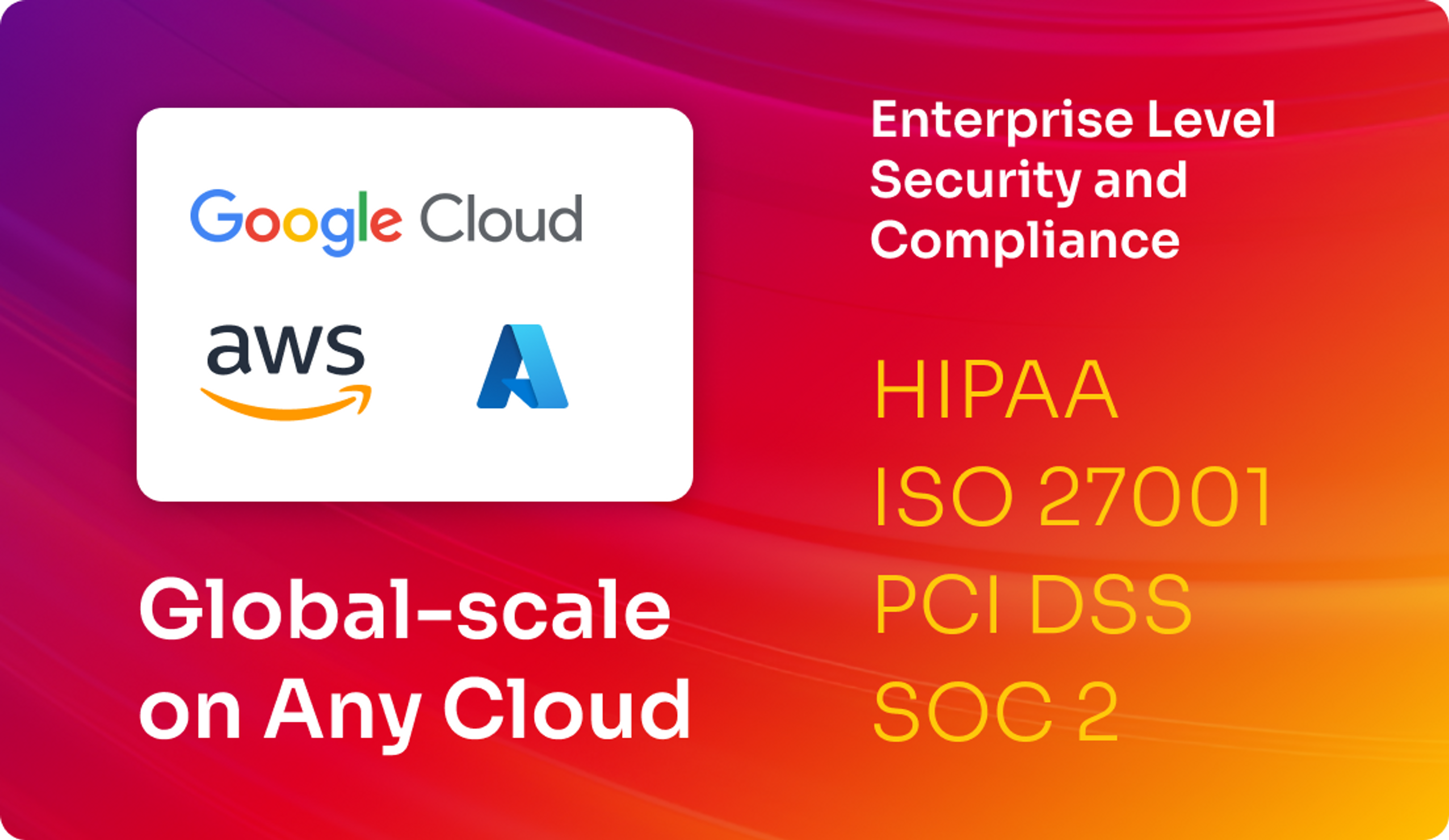
Shape the Wild
GenAI should be fun! An awesome developer experience for any JavaScript, Python, Java, and C++ dev to build production GenAI apps with LangChain, GitHub, Vercel, and the leading AI ecosystem partners.
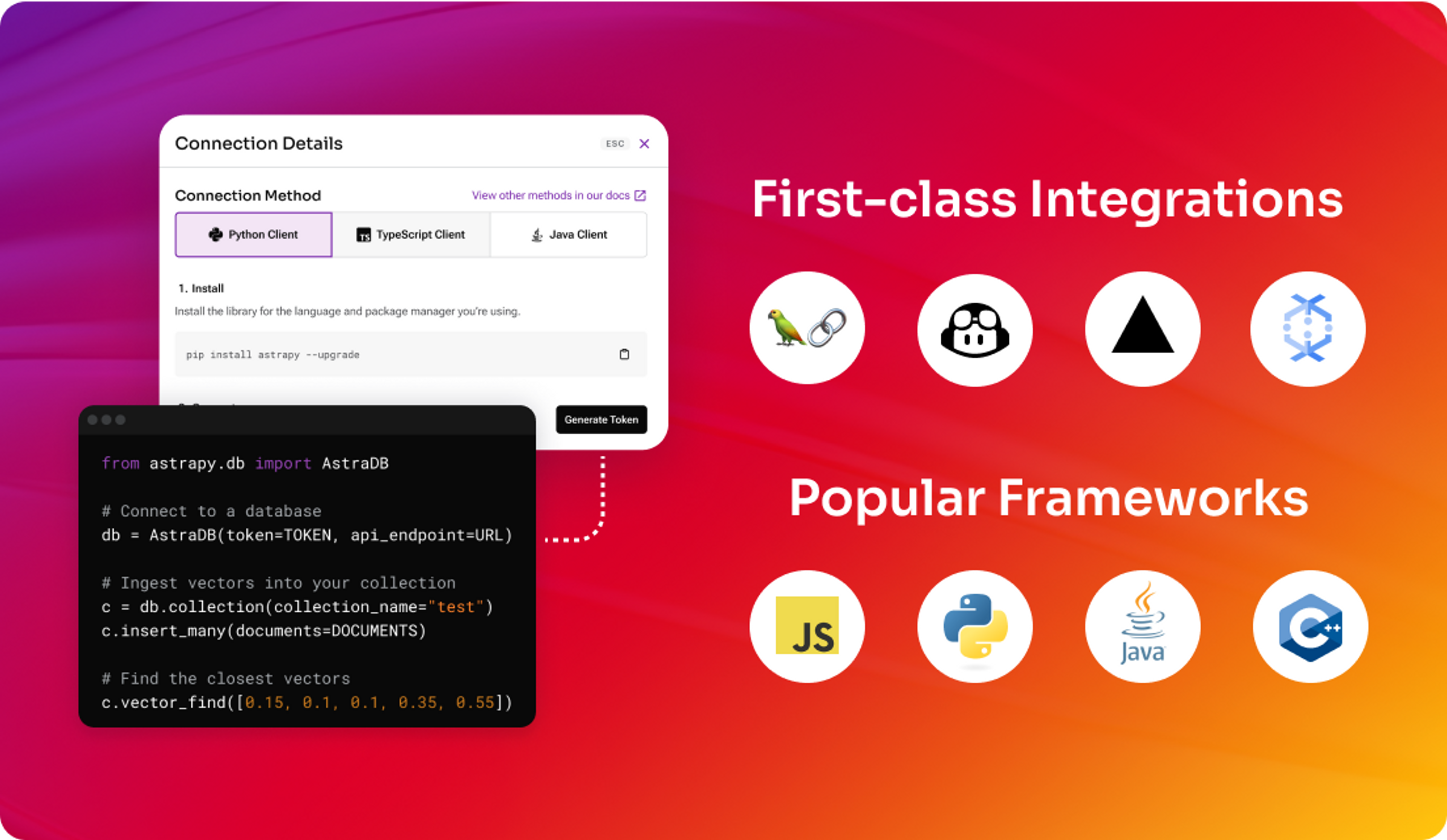
Developers
RAG Made Easier
An intuitive API and powerful integrations for production-level RAG and FLARE.
Install
Install the Astra library
pip install astrapy
Create
Create or connect to existing collection
# The return of create_collection() will return the collection collection = astra_db.create_collection( collection_name="collection_test", dimension=5 ) # Or you can connect to an existing connection directly collection = AstraDBCollection( collection_name="collection_test", astra_db=astra_db ) # You don't even need the astra_db object collection = AstraDBCollection( collection_name="collection_test", token=token, api_endpoint=api_endpoint )
Insert
Inserting a vector object into your vector store (collection)
collection.insert_one( { "_id": "5", "name": "Coded Cleats Copy", "description": "ChatGPT integrated sneakers that talk to you", "$vector": [0.25, 0.25, 0.25, 0.25, 0.25], } )
Find
Find documents using vector search
documents = collection.vector_find( [0.15, 0.1, 0.1, 0.35, 0.55], limit=100, )

$300/year in free credit and no credit card required.

Tutorials and sample Generative AI apps with best practices.

Get started in minutes with Generative AI and RAG.