4 Ways Graph Technology Can Help You Prevent Fraud this Holiday Season
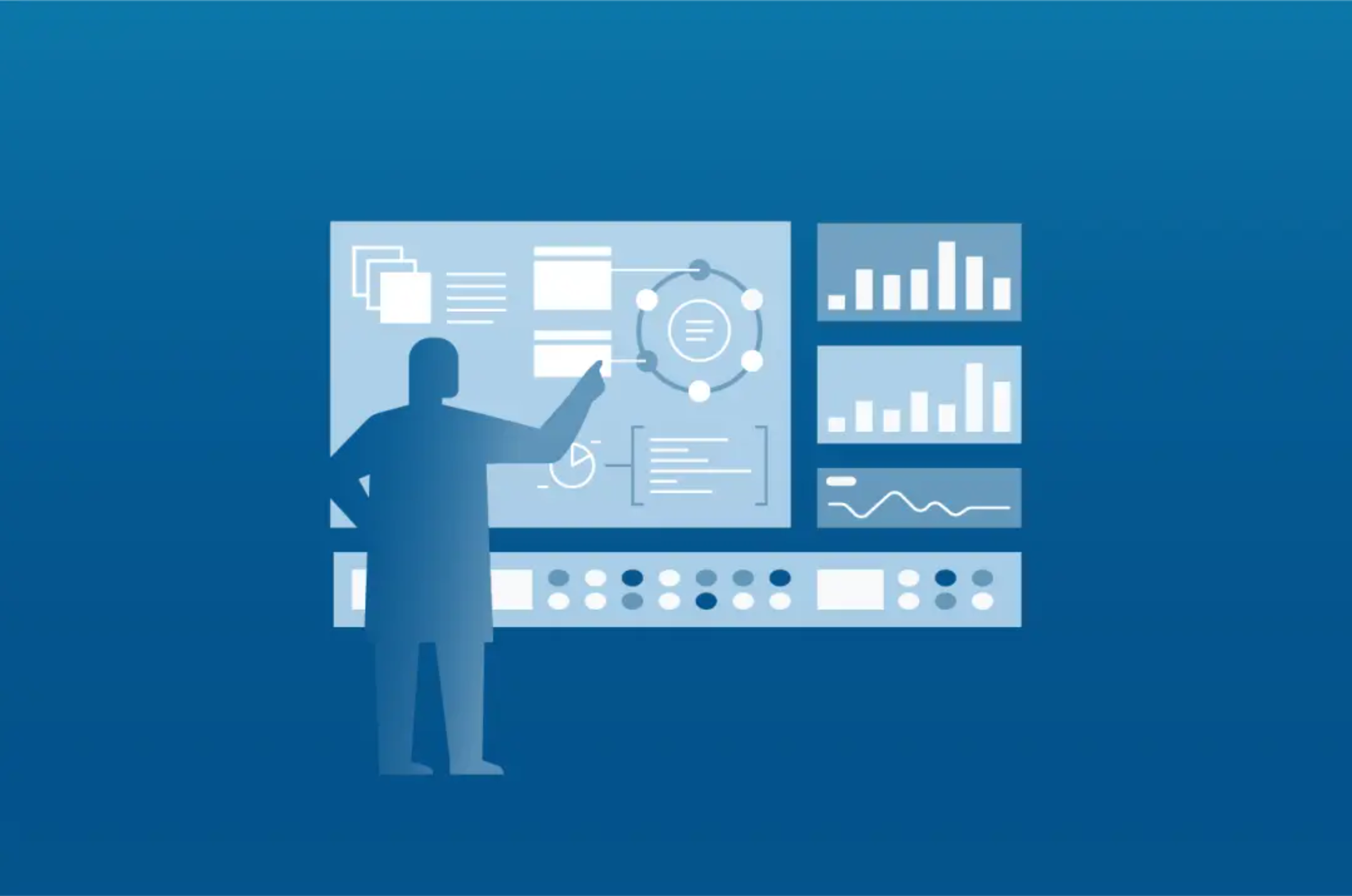
The 2019 holiday season is shaping up to be the biggest holiday season ever.
In 2018, the holiday season accounted for $707.5 billion in sales, including $150 billion in sales happening online or via non-store channels, according to the National Retail Federation (NRF). NRF expects those figures to increase this year, with online sales expanding as much as 12%—outpacing brick-and-mortar growth by as much as 5x.
Retailers are no doubt chomping at the bit to take advantage of this massive infusion of cash. But at the same time, an increase in sales corresponds to an increase in fraud attempts.
In fact, fraud attempts were expected to increase 14% last holiday season, with the volume of transactions increasing by 18% and their value increasing by 19%. Further, the average fraudulent transaction was expected to set retailers back $243.
We expect the 2019 holiday season to reflect similar numbers.
Few retailers have a big enough margin of error to absorb fraudulent transactions. And even if they do, no one wants to be the victim of fraud – if for no other reason than it hurts the bottom line or, at the very least, sucks up a lot of time.
The good news is that graph technology can help retailers prevent fraud this holiday season. Let’s take a look at four specific ways graph databases support fraud detection and prevention initiatives.
1. Identify more than just transaction data that are likely to be fraudulent
Historically, companies used relational database management systems (RDBMS) for fraud detection. But in today’s age of ubiquitous and disparate data, RDBMSs lack the ability required to not only identify but also connect the prolific volume of transactions to specific sources at scale.
In many instances, organizations would be required to partition data in order to detect fraud. As a result, they wouldn’t be able to analyze all of their data (100’s of millions to billions, even trillions of transactions over time) in a performant way, dampening the impact of their fraud prevention efforts.
With a scale-out graph database in place, however, organizations can analyze all of their data—including transaction data, demographic data, help desk information, shopping cart data, historical trends, and more—to determine which kinds of transactions are most likely to be fraudulent, by whom and even surface crime rings by identifying connected patterns between multiple accounts and fraudulent transactions.
A graph data is modeled in such a way that machine learning algorithms can be implemented more efficiently against that data to consistently improve fraud detection as well as prevention abilities.
2. Set rules that ensure transactions are within normal boundaries and patterns of behavior
For example, in traditional fraud detection systems transactions over a certain threshold are flagged and need to be signed off on – let’s say you’re a retailer that does the bulk of your business in transactions that are $100 or less.
Recall that statistic about how the average fraudulent transaction is expected to be north of $240. Using a graph database, you can not only set rules that require approvals or triggers for every transaction that’s over $100, but you can also identify trends in fraudulent transactions to the same store, cashier, customer, demographics, location, etc. to continue the example.
With a graph database you can get higher value from your data by leveraging the relationships between your data.
Graph databases enable you to reduce the number of fraud instances you encounter by automatically surfacing fraudulent patterns of behavior - beyond just identifying single fraudulent transactions. Graphs can help you surface possible crime rings or centralized fraud patterns in real-time from your transaction data.
With DataStax’s graph database you can also run analytics across all your data; identify trends over days, weeks, months even years for specific elements like items selected, cashiers, stores, groups of buyers, etc. where fraudulent transactions repeatedly occur over set periods of time
3. Require customers to confirm first-time transactions from anywhere they are
Is this person really making a massive purchase from a vendor they’ve never bought anything from before and shipping it halfway across the world?
While it is definitely within the realm of possibility, such a transaction can seem a little suspicious.
Rather than letting these kinds of transactions go through without a hitch, with graph technology you can identify and set actions for transactions that aren’t within a customers usual buying behavior. You can require them to manually confirm the transaction, and the locations where items are sent, making sure the new addresses are legit.
4. Receive predictive notifications or insights before a fraudulent transaction occurs
If you want to take the super-hands-on approach to fraud prevention, you can create a rule that automatically notifies you when a transaction goes through. While this approach probably wouldn’t work for a massive, global company, a smaller shop that doesn’t do thousands of transactions a minute could benefit from automatic notifications.
What if you could get automatic notifications served up before fraudulent transactions occur, information based on past purchasing patterns. Consider the power of a graph database; it can efficiently work across related data sources so can surface insights or predictions for purchasing patterns for individuals as well as groups.
So, with the assistance of a graph database you can automatically freeze transactions in real-time, and buy time to investigate the purchase or groups of purchases to determine whether or not it’s actually valid, or if there is a bigger issue before it occurs.
Ready to make the most out of the holiday season?
The holiday season is a great time for retailers. There’s a reason we call it Black Friday, after all.
But it’s also a time for bad actors to commit fraud, hoping that the massive uptick in business is enough to cloak their ill intentions.
Just because fraud rises during the holiday season doesn’t mean you have to suffer because of it.
With the right database in place, you won’t have to.
For more information on the IT risks retailers face this holiday season—and what you can do to avoid them—check out this ebook.