Season 1 · Episode 6
Data Meshes: Big Data Architecture Becoming Distributed, Declarative and Domain Oriented with Zhamak Dehghani
Episode Guest
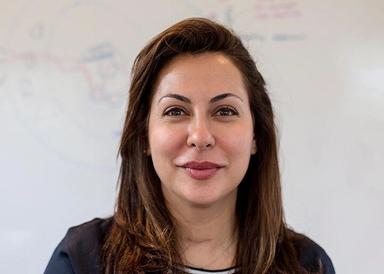
Zhamak Dehghani
Director of Emerging Technologies at ThoughtWorks North America
Director of Emerging Technologies at ThoughtWorks North America